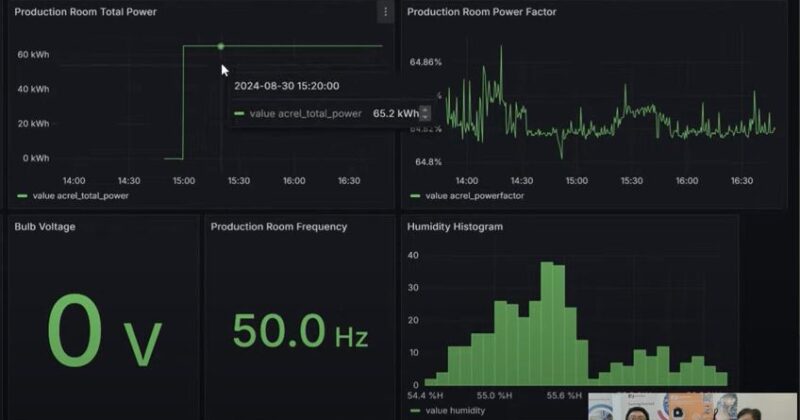
AIoT power management and optimization
Applying Industrial IoT and AI in power measurement and optimization
Introduction to Power Measurement with Industrial IoT and AI in Predictive Power ManagementIn today's rapidly evolving industrial landscape, effective power management is crucial for optimizing operational efficiency, reducing costs, and minimizing environmental impact. Traditional methods of power measurement and management often fall short in providing real-time insights, predictive capabilities, and the adaptability needed to address the complexities of modern industrial environments. This is where Industrial Internet of Things (IIoT) and Artificial Intelligence (AI) come into play, revolutionizing the way industries monitor, manage, and optimize their energy consumption.Power Measurement with Industrial IoTThe Industrial Internet of Things (IIoT) refers to the integration of interconnected sensors, devices, and systems within industrial settings, enabling the collection and exchange of vast amounts of data in real time. When applied to power measurement, IIoT allows for the continuous monitoring of electrical parametersâsuch as voltage, current, power factor, and energy consumptionâacross various equipment, machinery, and processes.Key benefits of...