The 6 Big Losses in OEE – Deep Dive into Unplanned Downtime
In the world of manufacturing, where efficiency defines competitiveness, OEE (Overall Equipment Effectiveness) serves as a critical performance benchmark. Among the six major losses that OEE identifies, Unplanned Downtime stands out as a particularly disruptive and costly factor — especially in modern, automated environments.
Let’s take a closer look at what unplanned downtime really means, its cascading effects on operations, and how manufacturers can tackle it more effectively using predictive AI-powered maintenance.
What Is Unplanned Downtime?
- Unplanned downtime refers to any unexpected stoppage of production caused by equipment failure or unforeseen malfunctions. Unlike scheduled maintenance or planned shutdowns, these interruptions catch production teams off guard — causing a sudden halt in operations and often requiring emergency repairs or adjustments.
With today’s increasing reliance on automation, the risks associated with unplanned downtime have multiplied. For manufacturers operating under lean principles or Just-in-Time (JIT) strategies, even a short delay can have a “cabin effect” — where a minor failure upstream leads to a full halt downstream. The ripple effects can include: - Missed delivery dates
- Contractual penalties
- Increased overhead from expedited shipments
- Bottlenecks in supply chains
- Lost customer trust and revenue
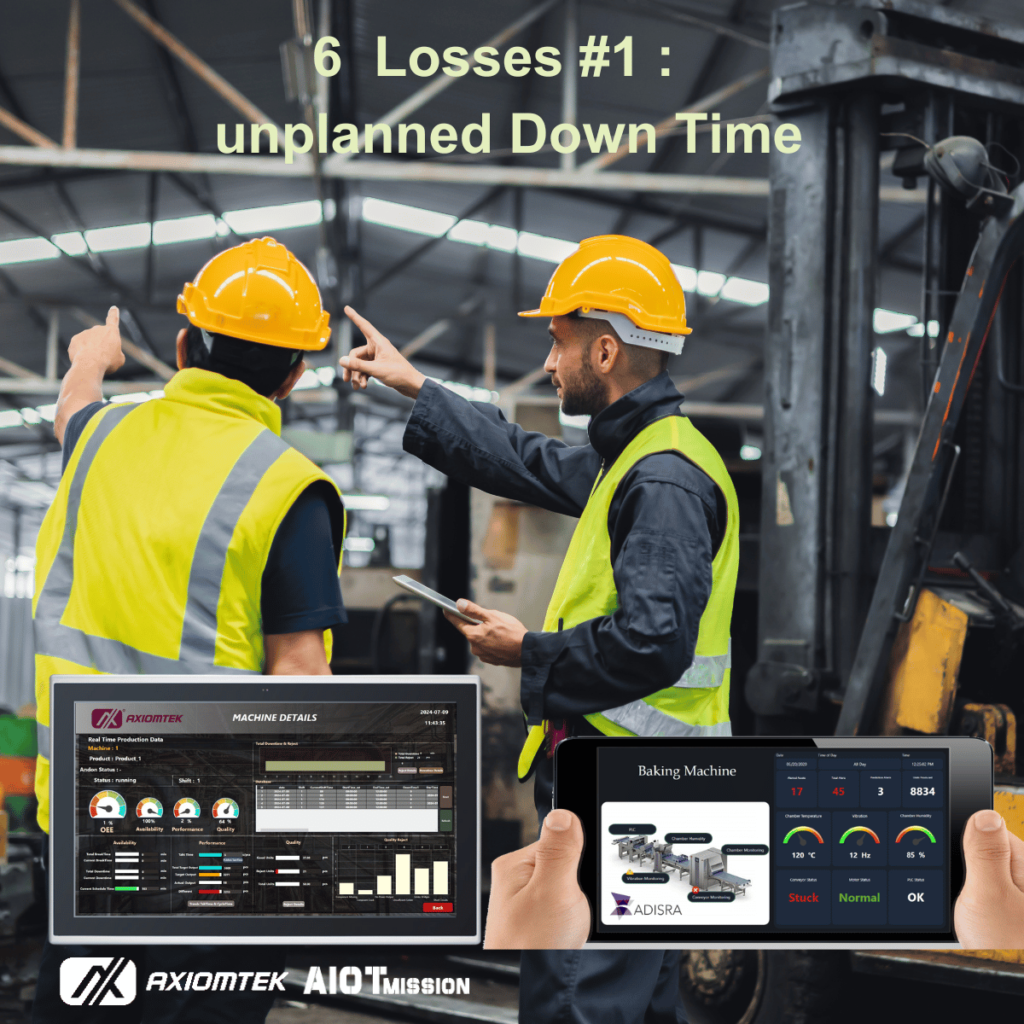
Preventive Maintenance: A Good Start, But Not Enough
Traditionally, manufacturers have relied on preventive maintenance as a first line of defense. This approach involves regularly scheduled inspections and part replacements based on estimated life cycles or runtime hours.
While helpful, preventive maintenance has limitations:
– It assumes components fail in predictable patterns
– It can lead to unnecessary part replacements, increasing costs
– It cannot detect sudden or hidden failures
– It depends heavily on human scheduling and observation
In short, it is reactive in nature — addressing problems on a calendar instead of in real time.
The Predictive Advantage: AI-Powered Maintenance
This is where predictive maintenance, driven by AI and real-time data, steps in to change the game.
Predictive AI continuously monitors equipment through sensors and connected systems. By analyzing patterns in historical data and detecting anomalies in machine behavior, it can forecast when a failure is likely to occur — and recommend action before it causes downtime.
Key Benefits:
– Minimized unplanned downtime through early detection
– Reduced maintenance costs by servicing only what’s needed
– Extended equipment lifespan
– Improved safety and compliance
–Increased OEE and overall operational efficiency
The Critical Role of Data in Predictive Maintenance
For AI-based predictive maintenance to work effectively, data is the foundation. Most manufacturing floors already have some form of SCADA (Supervisory Control and Data Acquisition) or automation systems in place that capture valuable machine data. However, the challenge lies in two key areas:
1. Data Formatting
Different machines and sensors produce data in various formats. Before any analysis can happen, this data needs to be standardized, cleaned, and structured for AI models to interpret accurately.
2. Data Integration
Systems on the shop floor often operate in silos — PLCs, sensors, SCADA, MES, ERP — each using different protocols. Integration is essential to give AI access to holistic, contextual information that connects events across machines and systems.
Our Approach: From Data Chaos to Predictive Clarity
We’ve partnered with Adisra SmartView to help manufacturers solve these very challenges.
Adisra SmartView offers a versatile, scalable platform that supports:
– Local and cloud-based deployments
– Rule-based and AI-based predictive models
– Real-time monitoring and alerting
– Integration with both legacy equipment and modern systems
– Support for the Unified Namespace (UNS) – ensuring future-proof data architecture
With this solution, manufacturers can rapidly move from traditional preventive strategies to a fully operational predictive maintenance model, without overhauling their existing infrastructure.
Final Thoughts: The Future Is Predictive
As the manufacturing industry embraces Industry 4.0, the shift from reactive to predictive maintenance isn’t just a technological upgrade — it’s a strategic necessity. Unplanned downtime is no longer a tolerable cost of doing business. With the right data strategy, tools, and AI integration, manufacturers can make downtime predictable, manageable, and in many cases, avoidable.
Key Takeaway:
Predictive maintenance turns real-time data into actionable foresight — reducing downtime, cutting costs, and driving smarter production decisions.
If your plant is struggling with unexpected failures or wants to explore how AI can transform your maintenance operations, let’s start the conversation.
A short video to discuss how AI improve production planning where CC Lee ( CEO and founder of AIoTmission) will be writing an article on the planned to Stop the #2 OEE losses.