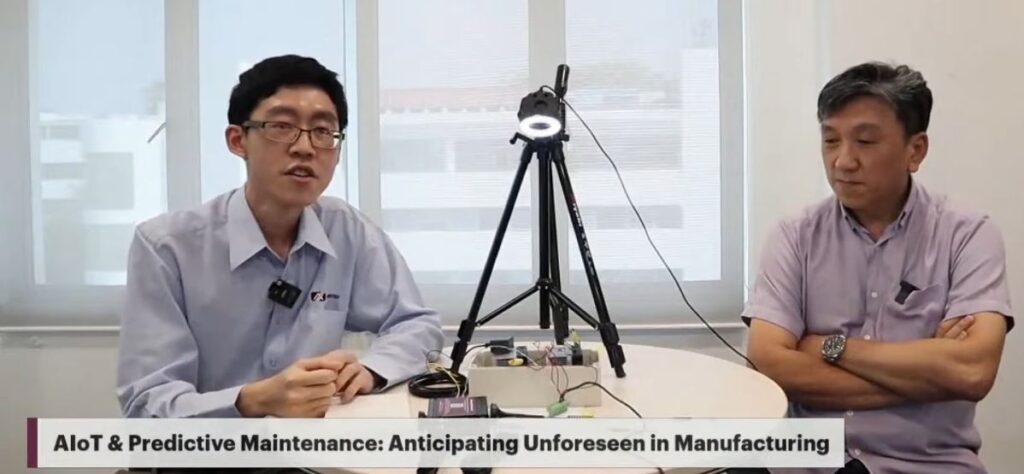
Live sharing on Predictive Maintenance with AIoT
During the live sharing on the Predictive Maintenance with AI and Industrial IoT, Tan Kien Leong has started with the brief introduction of how the maintenance process was carried out in the past and what are the limitation with the process of maintenance being performed in the old days. One of the recently practiced preventive maintenance is used to make a comparison to the newly introduced Prediction Maintenance.
Preventive maintenance (PM) and predictive maintenance (PdM) are both proactive approaches to maintaining equipment and systems, but they differ in methodology, technology, and application. Here are the main differences between the two:
- Definition:
- Preventive Maintenance (PM): Maintenance activities are performed at predetermined intervals or according to prescribed criteria, regardless of the actual condition of the equipment. It’s based on time or usage (e.g., every 10,000 miles or 1,000 operating hours).
- Predictive Maintenance (PdM): Maintenance is performed based on the actual condition of the equipment, as determined by monitoring and analyzing data from sensors, diagnostics, and analytics tools. The goal is to predict when a failure might occur and perform maintenance just in time to avoid unplanned downtime.
- Methodology:
- PM: Often follows manufacturer’s recommendations, historical data, or industry best practices.
- PdM: Relies on real-time data and condition-monitoring tools, such as vibration analysis, infrared thermography, ultrasound, and oil analysis.
- Technology Utilization:
- PM: Typically uses basic scheduling tools or CMMS (Computerized Maintenance Management Systems) to keep track of maintenance tasks.
- PdM: Utilizes advanced technologies, including IoT sensors, machine learning algorithms, and data analytics to predict equipment failures.
- Cost:
- PM: Can sometimes lead to over-maintenance, resulting in unnecessary costs and potential wastage of parts and labor.
- PdM: Often results in cost savings by preventing unexpected breakdowns and minimizing maintenance activities to only when necessary.
- Waste:
- PM: There’s potential waste as parts might be replaced or maintained even if they’re still in good condition.
- PdM: Minimizes waste because components are only serviced or replaced when their condition indicates a need.
- Downtime:
- PM: Maintenance shutdowns are scheduled, but there’s still a risk of unexpected failures between scheduled maintenance activities.
- PdM: Reduces unplanned downtime since maintenance is performed when there’s a clear indication of deterioration.
- Implementation Complexity:
- PM: Easier to implement, as it’s based on set schedules or intervals.
- PdM: More complex due to the need for advanced tools, sensors, and data analysis capabilities.
- Flexibility:
- PM: Fixed schedule, less flexibility.
- PdM: Highly flexible and adjusts based on actual equipment condition.
IIoT edge gateway & 4G router gateway demo with Vibration sensors
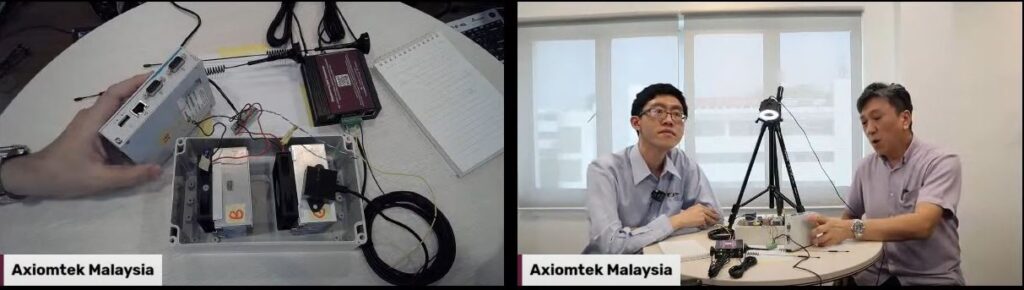
- Vibration Sensors: Vibration data can provide insights into the mechanical condition of rotating machinery. An increase in vibration levels or unusual vibration patterns can indicate misalignments, unbalance, wear, or other issues.
- Temperature Sensors: Temperature measurements can indicate overheating or abnormal thermal behavior in equipment components. Sudden temperature changes might point to faulty parts or impending failures.
- Pressure Sensors: Pressure measurements are crucial for systems that involve fluids or gases. Abnormal pressure levels can signify leaks, blockages, or other issues.
- Current and Voltage Sensors: These sensors are particularly relevant for electric motors and other electrical equipment. Changes in current and voltage patterns can indicate electrical faults or imbalances.
- Humidity Sensors: Humidity data is essential in environments where moisture can affect equipment performance. High humidity levels might lead to corrosion and damage.
- Speed and Rotation Sensors: These sensors monitor the speed and rotation of moving parts. Deviations from expected speeds can indicate mechanical problems.
- Proximity Sensors: Proximity sensors detect the distance between the sensor and a target object. They can be used to measure clearances, displacements, and alignment issues.
- Strain and Load Sensors: These sensors measure mechanical strain and load on structures. They are used to monitor stress levels in equipment components.
- Acoustic Sensors (Sound): Acoustic data can help identify abnormal sounds or noise patterns that might indicate mechanical issues, such as friction or wear.
- Gas Sensors: Gas sensors are relevant for detecting leaks or abnormal gas concentrations. They are used in systems involving gases or chemical processes.
- Position Sensors: Position sensors track the position of moving parts. They can detect misalignments or deviations from expected trajectories.
- Force Sensors: Force sensors measure applied force or load on a surface or component. They can help monitor stress levels and potential failures.
- Light Sensors: Light sensors might be used in situations where changes in light intensity or frequency can indicate equipment issues.
- Image Sensors (Cameras): Cameras can capture visual information for visual inspection and anomaly detection. They are especially useful for identifying visible defects or irregularities.
- Tilt and Inclination Sensors: These sensors detect changes in inclination or tilt. They can be used to monitor the stability and orientation of equipment.
Vibrational data being fed to the Aiot edge connect cloud with AI ML on the data
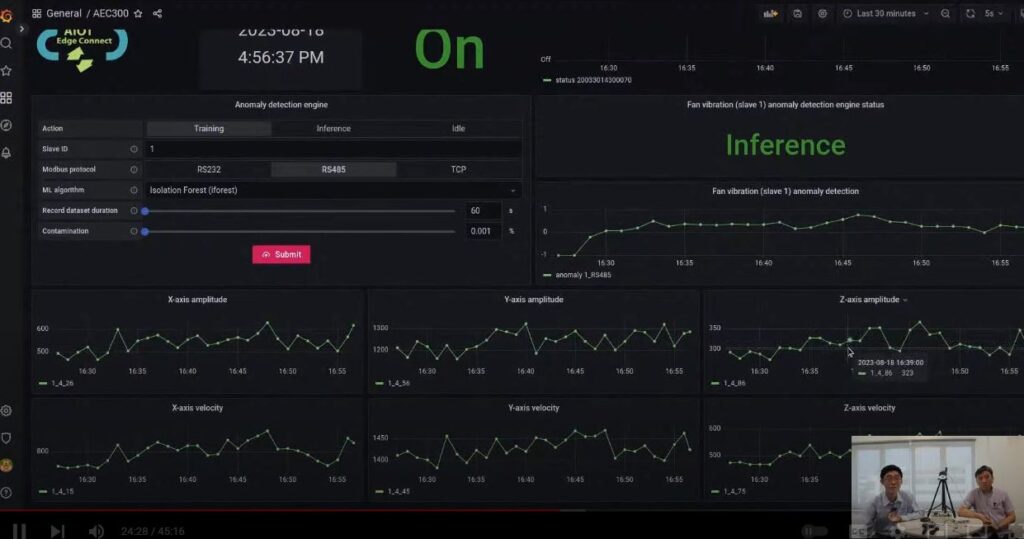
In the demonstration, the Vibrational data from two rotating fan were used as a source of vibration signal being captured. The data is collected via the 4G router gateway ( AEC310) and optional we can use Axiomtek IIoT edge gateway.
Three-axis vibration measurement
Frequency range: 10 Hz to 1K Hz
Amplitude range: ±8g
Data Format:
-Acceleration(mg): RMS,
Maximum, P-P
-Velocity(mm/s): RMS
-Top 10 spectrum values(Amplitude/Frequency)
To Watch the live session, please click the link below:-