Integrating AI and IoT for optimal IR4.0 performance
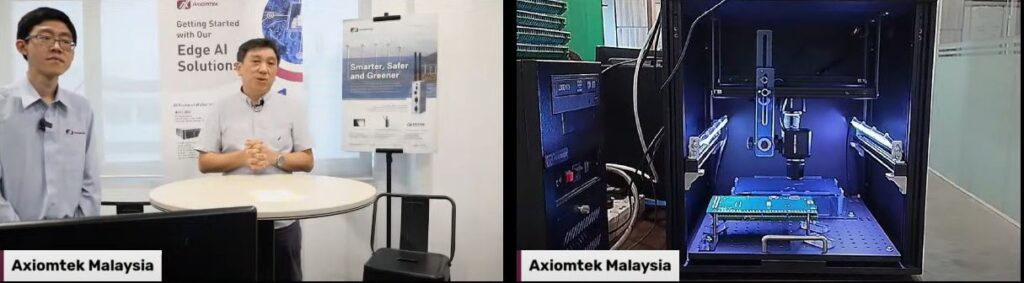
This session of ” Sembang AIoT” talk more about the integrating IIoT and AI for optimal performance of industry 4.0 performance.
while IR4 principles lay the foundation for a connected, transparent, and autonomous industrial environment, it is the integration of AI and IoT that actualizes and optimizes these principles to achieve the full potential of Industry 4.0.
Industry 4.0 (IR4) is the current trend of automation and data exchange in manufacturing technologies, encompassing cyber-physical systems, the Internet of Things (IoT), cloud computing, and cognitive computing. Here are the four main design principles of Industry 4.0:
- Interconnection
- Decentralization
- Data Transparency
- Technical Assistant
The four fundamental design principles provide a clear framework for Industry 4.0’s goals and future direction. Interconnection and Information Transparency are intrinsically linked to the Internet of Things (IoT), emphasizing the significance of connectivity. Data collected from sensors, machines, and controllers necessitates the use of IoT devices to maintain continuous connection and facilitate real-time data transfer to the cloud. This ensures consistent and transparent data throughout various processes.
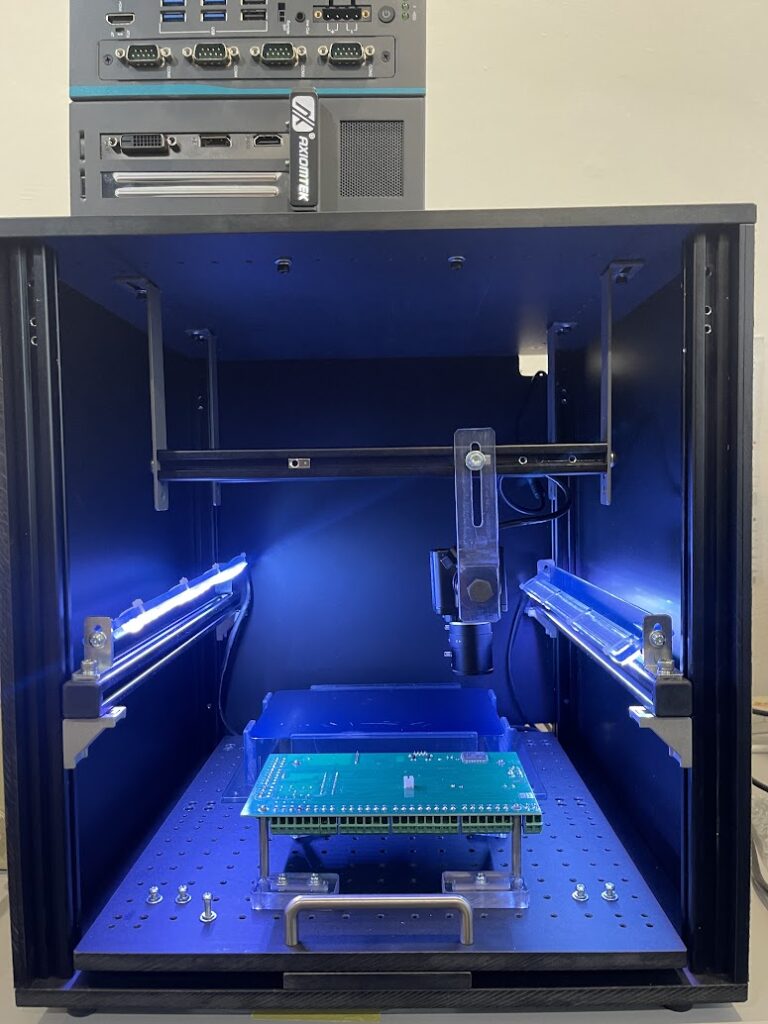
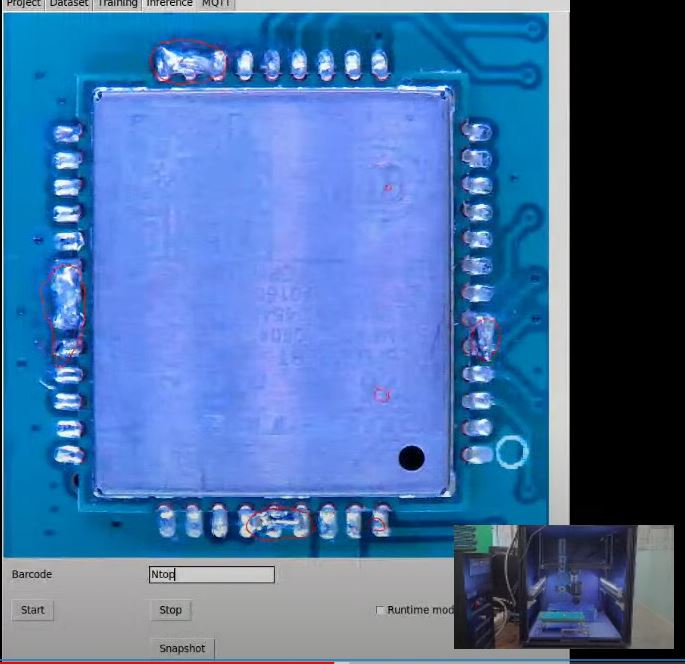
https://axiomtek.my/product/ipc962-525-axiomtek-industrial-pc/This session is further enhanced with the properly set demonstration on the unsupervised Deep learning anomaly detection system. Axiomtek’s Fanless industrial PC is used in this deployment of demo. In order to speed up the inference process, one of the Entry level GPU is used to keep up with performance.
The unsupervised deep learning AI in this model do not require any intervention on the dataset that are used. Good dataset or samples are fed and with minimum work on the result that we need to achieve. We took one of the good example of Printed Circuit Board ( PCB) visual inspection process where the time required to check on the PCB takes about 45 seconds per board. But with Vision anomaly AI, It took only 2 seconds on the entire PCB with 4 to 6 cameras depending on the size of the PCB.
The picture on the left show the visual image of marked red AI spotted unwanted soldered joined and those images can be marked and stored as part of the defect listing.
Different zones of defects can be recorded for the next process of rework.
Joining our Free AIoT Workshop for Free!
We are offering a Free hours workshop ( valid till end of 2023)
Scan the QR code below to register!
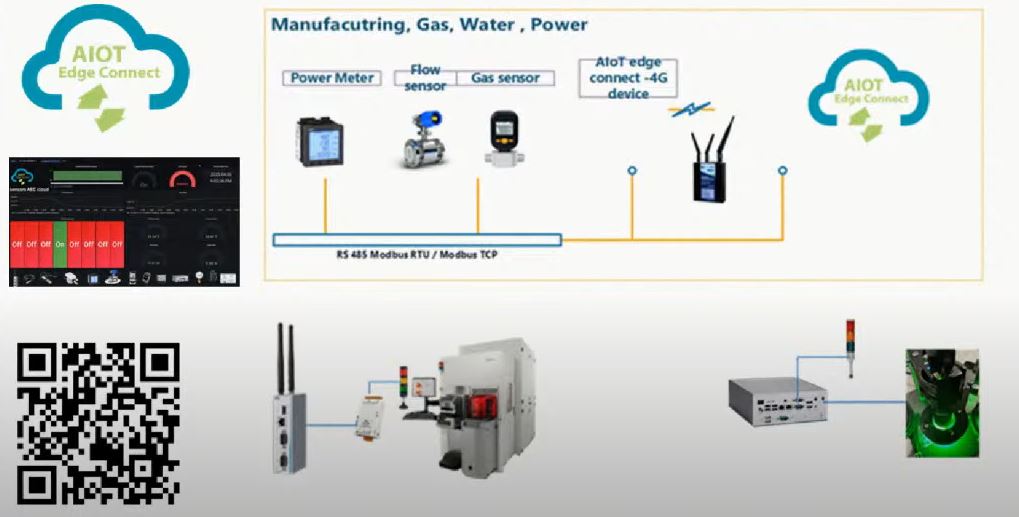