6 key losses -The start up Defects
Understanding Start-Up Rejects in OEE:
A Hidden Quality Drain
What Are Start-Up Rejects?
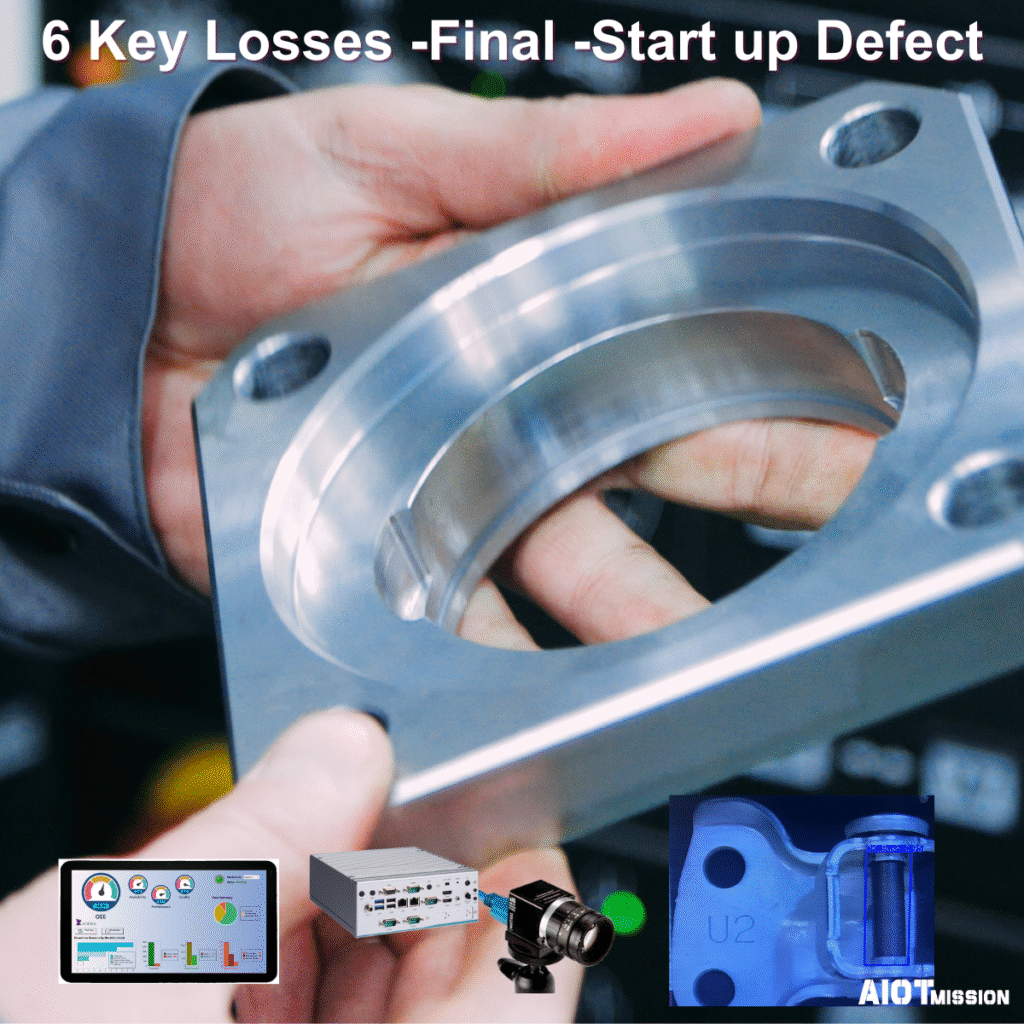
Why Start-Up Rejects Matter
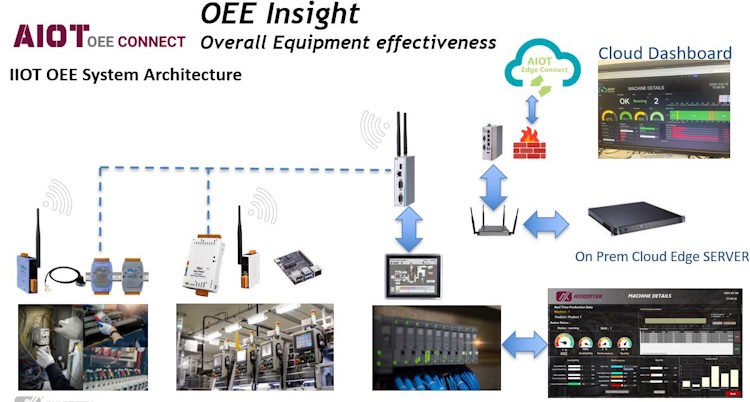
Why Start-Up Rejects Matter
Detecting and Tracking Start-Up Rejects
-
Time-based tagging: Mark the first X minutes or Y units after start-up as “start-up phase.”
-
Sensor-triggered events: Use PLC signals or IoT device triggers to identify exact machine start points.
-
Manual entry: Operators log the first-good part or timestamp, though this is more error-prone.
Analyzing Root Causes of Start-Up Rejects
-
Incorrect parameter presets
-
Residual material contamination
-
Mechanical drift or wear
-
Improper tool alignment
-
Inadequate pre-startup checks
Enhancing Start-Up Quality with AI and Analytics
-
Predict when a machine is ready for optimal output
-
Trigger alerts if parameter drift is detected
-
Adjust warm-up profiles dynamically
-
Correlate reject types with specific operational anomalies
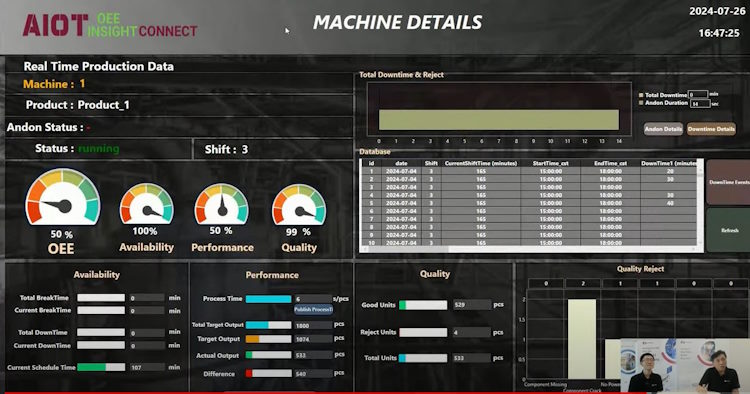
AIoT OEE Insight Connect is a groundbreaking, next-generation solution designed to deliver deep, real-time visibility into manufacturing performance. More than just an OEE (Overall Equipment Effectiveness) tracker, it offers a comprehensive and intelligent approach to uncovering the true drivers behind production efficiency — empowering smarter, faster decisions.
At its core, AIoT OEE Insight Connect excels in high-fidelity data acquisition, capturing critical information from:
-
Sensors and machines,
-
Controllers and PLCs,
-
Operator and human inputs.
This multi-layered data integration ensures a true 360° view of what impacts OEE, drilling down to the root causes that traditional systems often miss.
Hybrid-Cloud Flexibility: Modern, Secure, and Scalable
Harnessing the latest advances in cloud technology, AIoT OEE Insight Connect seamlessly streams all operational data to a secure, intuitive cloud dashboard.
At the same time, it gives manufacturers the freedom and flexibility to deploy the system fully within their on-premises infrastructure if preferred — maintaining control, security, and customization according to operational needs.
Actionable Intelligence with Generative AI
Where this solution truly stands apart is in its analytical intelligence:
-
Advanced reporting and analytics uncover patterns, bottlenecks, and optimization opportunities.
-
Generative AI integration goes a step further, automatically generating actionable recommendations and task lists designed to continuously enhance process performance.
By bridging real-time shop floor insights with AI-driven action plans, AIoT OEE Insight Connect ensures that continuous improvement becomes an embedded, automated process — not just an aspiration.
Key Benefits at a Glance:
-
Comprehensive OEE measurement and deep root-cause insights
-
Real-time, flexible access via cloud or on-premise deployment
AI-powered action plans for continuous process optimization
-
Seamless integration with existing industrial systems and infrastructures
AIoT OEE Insight Connect is not just a tool — it’s your partner in building a more efficient, agile, and intelligent manufacturing future.